What is patient analytics?
Patient analytics, the use of data analytics in the healthcare sector, involves automating data collection and analysis to provide better healthcare. Patient analytics can redefine healthcare efficiency and costs—not just for the medical sector—but for individual patients too. This analysis helps healthcare professionals make better decisions.
The impact of patient analytics on the medical industry can be huge, such as lowered costs in providing quality healthcare, customizing treatments for individuals, or improving the processes of pharmaceutical companies. Patient analytics can be at the macro and micro levels, improving the quality of medical care and reducing costs for patients.
With patient data analytics, healthcare professionals can improve medical care and the overall patient journey. Patients go through a long cycle of care, starting at diagnosis through the end of treatment. This entire journey—from admittance to diagnosis to treatment to discharge—is the patient experience. Artificial intelligence (AI), a form of machine learning (ML) utilized in data analytics, helps review patient experience data quickly and accurately.
Analyzing patient experiences helps the medical industry provide better services and improve their offerings. Hospitals can aggregate patient feedback through several sources: service calls, online forms, rating systems, interactive voice responses systems (IVRs), and much more. These data sets can help health professionals attain high-quality insights about their patients’ specific concerns, such as doctor availability, wait times, communication, and medications.
The role of patient analytics in patient care
Patient analytics has several use cases across the medical sector. Analyzing patient data can hugely change outcomes in a positive way.
General health management of the population
Several data analytics technologies are currently in use across the healthcare industry. This has helped the healthcare industry develop Population Health Management (PHM) services. Through PHM services, the goal of analytics technology in healthcare has expanded from just providing adequate treatment and faster response times to predicting and preventing illness.
With the right kind of predictive analytics tools, the medical industry can spot potential risk patterns in a patient. This gives doctors a chance to address the illness before it reaches a complex end-stage. Predictive analytics in healthcare is able to not only bring down the cost of treatment but also to save lives through early interventions.
Health tracking for preventive care
Utilizing predictive analytics, the medical industry can foresee potential chronic health risks. With quality training data, powerful analytical tools can constantly work on analysis models. These models will delve into medical records and find patterns that can predict the risk levels associated with certain diseases. These models can be built up to create a healthcare tracking system that anticipates the needs of patients and offers them better services.
With such technology, a patient’s health is constantly monitored across various parameters, enabling preventive health care methods for early detection and treatment. It also ensures the right care is given at the right time.
Advancement of the health industry
Besides the patient benefits, analytics technology also goes a long way to developing future ready business intelligence (BI) solutions. Large amounts of the most complex medical data can be analyzed to develop improved treatment facilities customized to every patient’s medical record.
Challenges with patient analytics
As with any form of data analytics, patient analytics has its share of challenges.
In the US, electronic medical records (EMRs) and electronic health records (EHRs) are part of the challenge. EMRs are a digital medical chart restricted to a single practice or hospital. EHRs are a more holistic look at the health of the patient.
Big data is constantly innovating and finding ways to improve real-time data collection, capture information, analyze data, and create usable insights, using a range of visualizations.
EHRs lack uniformity such as in government product labeling, capabilities, and the clinical terminology for them. The challenge is to work on accessing in-depth actionable insights from vast, duplicated data.
Storing vast amounts of data
Data access and storage poses a major challenge to patient analytics. As methods of data storage have expanded to include cloud storage alongside traditional methods of on-premises storage, healthcare organizations must choose a data storage and access system that aligns best with the privacy and security regulations they must adhere to.
On-site servers can be an expensive proposition, not just to set up, but to maintain and scale out. Cloud-based storage has become popular because of its lowered costs and increased reliability. The medical sector must choose a cloud partner that implements all compliance and security regulations. Some services providers offer a hybrid approach to data storage. The right kind of service provider will be scalable and offer data access and storage that suits the organization’s regulatory and security requirements.
Cleaning data for accuracy
Data is often stored in its raw format and needs to be cleansed for accuracy and consistency and checked for relevance. The process of cleaning can be manual or automated. Manual cleaning can subject the data to higher chances of human error and waste valuable resources. Larger companies often apply high-end ML-based techniques to data cleaning. This reduces the time needed to clean data, increases data quality, and lowers expenses.
Creating a unified format
Patient data can be highly varied, considering the dynamic nature of ailments and the information recorded for each one. Traditional formats may not easily capture all the information required for analysis. There have been efforts to standardize information in systems such as the current procedural terminology (CPT) or international classification of diseases (ICD) codes. However, each of these codes comes with limitations that often impede data analysis.
Inaccurate data collection
When patient data is moved into other forms, there is a chance it is recorded inaccurately. This can be because of an inefficient data setup, complex information flows, or a lack of training—all contributing to poor data quality.
Processing of images
Data, especially in the form of images, can be misinterpreted from medical records. Medical images can show anatomy incorrectly or appear irrelevant to a particular case. Therefore, healthcare providers need to ensure medical images are processed to reduce noise, enhance contrast where needed, and have quality-adjusted parameters to overcome any discrepancies.
Dealing with security breaches
Medical organizations are often the victim of security breaches and hacking. Considering the sensitivity of the information in such organizations, data security is a priority. Many technical safeguards have been put in place to protect information. These rules help organizations with the right way of storing their data, transferring it securely when needed, and installing authentication protocols in place for data access and auditing. Security measures—such as the latest anti-virus software, necessary firewalls, and encryption methods—are needed to protect sensitive data. Cyberthreats tend to get more sophisticated with time and have to be constantly evaluated and protected against.
The need for quality metadata
For good data governance, healthcare providers need updated metadata for all stored data. Metadata holds information such as the time created, who used it, and its purpose. This makes way for data analysts to easily recall earlier queries and set up a benchmarking standard. Quality metadata increases data usability and prevents data silos. Organizations must make sure that metadata is automatically recorded and entered on each piece of data, as this is essential to quality, organized data.
Lack of interoperability between data sets
With the right kind of metadata in place, organizations can begin working with their data and gain needed insights. But, with inconsistent data sets, their query tools cannot gain complete access to their data repository. All data set components must be connected and accessible to generate a complete picture of a patient’s health. Structured query language (SQL) is one solution that can help query massive data sets.
Accurate visualizations
Despite having quality data on hand and great analysis tools, results are not automatically presented in a visually appealing manner. Using a wide range of visualization tools such as charts, histograms, and interactive maps can make data easily understandable. Achieving this level of accuracy in visualization can be challenging and requires in depth understanding of different charts and their uses, as well as an intuitive dashboard set up.
Inconsistent data sharing
Patients don’t necessarily receive all their care from a single place. Therefore, data collection can vary, which can be confusing for data management teams. Sharing this information is important to ensure continuity of patient care. If there is some disparity in data storage parameters, it can lead to a block in information flow, resulting in missing information and lowered data accuracy.
For patient analytics to be optimal and useful, organizations must address all these challenges. With the right data management and analytics strategy in place, organizations can gain a reliable and trusted data ecosystem. This will ensure that data analytics is then timely, relevant, and useful to all stakeholders.
Patient analytics can ensure better outcomes in many ways:
- Avoiding drug interactions with patient prescriptions
- Providing continuous care through the entire healthcare system
- Increasing holistic treatment options from shared historical health information
- Identifying high risk patients effectively
- Diagnosing illnesses correctly with ML-based models
- Lowering costs for medical providers with more accurate treatments
- Utilizing staff better and predicting levels of nurse requirements
The benefits can be massive. However, there are significant barriers to effective patient analytics:
- Patient data is highly sensitive, and its protection has to be a top priority. Medical professionals must ensure this data remains secure.
- Organizations need to address and resolve any biases that exist within their analytical algorithms. Since algorithms are written by humans, they are prone to error and bias. Considering factors like race, gender, and geography all affect health care access, it is possible that information regarding minority groups does not get recorded correctly. ML-based algorithms learn from the data provided to it.
- The range of data types, software, legacy systems, and architecture means that one single platform is unlikely to meet an organization’s needs.
To ensure your healthcare data has a positive impact on your patients, be sure to use top-notch analytical methods and employ data privacy best practices.
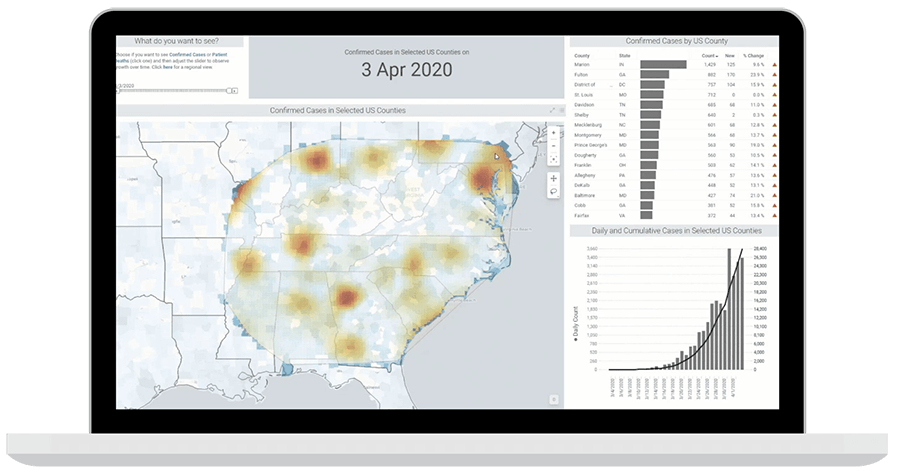