What is predictive analytics?
Predictive analytics, a branch of advanced analytics, is the method or technique of using data to model forecasts about the likelihood of potential future outcomes in your business. Predictive analytics uses historical and current data combined with techniques such as advanced statistics and machine learning to model unknown future events. It is generally defined as learning from past collective experience of an organization to make better decisions in the future using data science and machine learning.
Predictive analytics allows organizations to predict customer behavior and business outcomes, using historical and real-time data to model the future. Furthermore as a subset of this activity, predictive modeling is the process of creating and maintaining models, testing and iterating with existing data, and applying embedded models within applications.
Predictive analytics allow for identifying patterns contained in data to assess risks or opportunities for your business, addressing important business questions like: which machine needs maintenance? Which product should I recommend now? And who’s in danger of going into cardiac arrest? Essentially, you can use predictive analytics to forecast confidence levels of events based on very defined conditions and parameters.
What are the main benefits of predictive analytics?
Business managers are constantly making decisions that affect every aspect of their business: operations, production, personnel, marketing, and finance. Some decisions are purely day-to-day operational ones, some are tactical responses to competitive market moves, and others are long-term strategic decisions. They all affect the returns to stakeholders that could determine whether the business is able to raise new capital in the stock market or acquire new donors and contributors to finance its operations and new product development efforts. All of these decisions are critical.
The basis for these critical decisions are insights. Business managers cannot make these decisions in a vacuum. But where do these insights come from? The only true source of insights is data. Data, however, must be analyzed and presented in meaningful ways in order to yield the required insights. Raw data isn’t very useful. Businesses are awash in data that come from numerous and diverse internal and external sources, including manufacturing processes, supply chain pipelines, online and traditional transactions, sensors, social media, company and product reviews, government and trade association reports, and so on. All of this data also comes in different forms such as text, images, audio, videos and, of course, numbers. Management’s problem is how to extract from all this data the actionable, insightful, and useful information it needs (or its customers need) for their decisions.
Businesses must ultimately compete on data, and the pathway into the data is analytics. Analytics has three components:
- Data exploration and visual analytics: For identifying new insights and unseen problems and issues
- Data science and machine learning: To model and predict potential outcomes from the business’s and the markets’ actions
- Reporting: For distributing information to help stakeholders so that optimal decisions can be made
While either embarking on a new data analytics endeavor or enhancing an existing one that is outdated and insufficient for changing environments, how does management maneuver through all of the issues and complexities that encompass data analytics? You need to know what to consider and understand how the pieces fit together to produce the right insights for intelligent business decisions.
Deciding between data exploration and visual analytics, data science and machine learning, and reporting is complex. What questions should you ask and what answers will help guide you to the right choice?
The Analytics Spectrum shown in the figure below is a series of questions with guiding answers for you to consider that can help you decide what you need for your business.
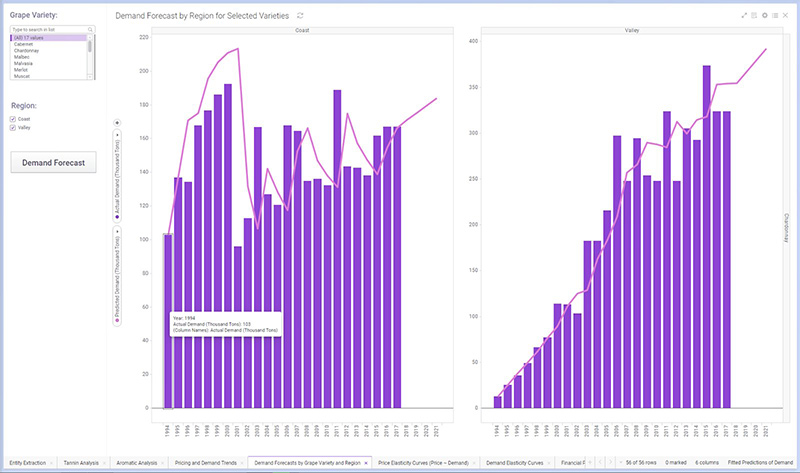
The Analytics Spectrum shown in the figure above is a series of questions with guiding answers for you to consider that can help you decide which type of analytics that you need for your business.
Predictive analytics goes beyond simple descriptive analytics which are the basics that most companies utilize today. Descriptive analytics can only tell the business what has happened. To predict and uncover insights about the future of the business, you need predictive analytics. Those insights can prove extremely valuable in reducing risks, optimizing operations, and increasing profits. Even better, predictive analytics help businesses solve complex problems with predictive models and find new opportunities for business success.
What are some business applications of predictive analytics?
Predictive analytics allows businesses across different industries to seize opportunities by using both past and present knowledge to predict what might happen in the future. Fraud detection, for example, relies on predictive analytics to identify patterns in the data that indicate fraud, spot anomalies in real time, and prevent future threats. Predictive analytics can also help optimize operations by forecasting trends that may impact resources and marketing efforts by predicting potential customer behaviors. Finally, predictive analytics is often used in manufacturing to evaluate assets, implement predictive maintenance, and reduce costs associated with machine downtime.
- Anomaly detection
- IoT and engineering
- Energy: production surveillance, drilling optimization
- Predictive maintenance
- Manufacturing: yield optimization
- Financial services
- Trade surveillance
- Fraud detection
- Identity theft
- Account and transaction anomalies
- Healthcare and pharmaceutical
- Patient risk assessment: cardiac arrest, sepsis, surgery infection
- Patient vital signs monitoring
- Medication tracking
- Customer analytics
- Customer Relationship Management: churn analysis and prevention
- Marketing: cross-sell, up-sell
- Pricing: leakage monitoring, promotional effects tracking, competitive price responses
- Fulfillment: management and pipeline tracking
- Competitive monitoring
How do predictive analytics work?
Predictive analytics rely heavily on machine learning (ML). ML is a combination of statistics and computer science that is used to create models by processing data with algorithms. These models can recognize trends and patterns in data that are generally deeper in sophistication than just visual data discovery methods alone. Using data from diverse sources (for example, the Internet of Things (IoT), sensors, social media, and an array of devices), machine learning processes that data through sophisticated algorithms and builds models for identifying and solving a problem and making predictions.
A model could be as simple as describing the impact on one component of manufacturing (for example, “If material supplies delivery are delayed one hour, shipments of final products are delayed one week”). It could also be something more complex, involving multiple impacts due to multiple concurrent issues. Machine learning can wade through troves of data and take into account complex interactions to create models that human knowledge workers cannot accomplish. Machine data is therefore commonly used for images, video, and audio analysis.
Predictive analytics also rely on data science, which is a more encompassing concept that just ML. Data science combines statistics, computer science, and application-specific domain knowledge to solve a problem. In a business setting, it combines machine learning methods with business data, processes, and domain expertise to solve a business problem. Basically, it provides predictive insights to decision makers.
We can embed a model to predict a likely outcome or provide an optimized solution to changes in process parameters directly within business processes. A model provides a competitive advantage because it does the following:
- Enhances capabilities
- Speeds decision making
- Processes large amounts of disparate data types
- Generally lowers the costs of operations
- Generates new revenue streams
- Leads to differentiated products and service offerings
The embedding of a predictive model in business processes is the joint goal of data science and machine learning.