What is healthcare analytics?
Healthcare analytics is the use of a combination of real-time and historical data to predict trends, reveal actionable insights, and achieve medical advances. It allows healthcare professionals to find scope to improve operations, patient engagement, care delivery and clinical outcome.
The vast amount of data collected through healthcare analytics helps organizations in the healthcare sector with fact-based decision making–helping to improve planning, management, measurement, and learning.
This form of data collection gives insight at both macro and micro-levels of healthcare and can show trends to help improve patient care quality, diagnosis, improving outreach, and better management of the spread of diseases.
Is healthcare analytics necessary?
The healthcare industry across the globe is experiencing a fundamental transformation as it moves from a volume-based to a value-based business model. The ever-increasing demand of consumers for improved healthcare quality has put this service delivery sector under pressure to provide better outcomes.
Healthcare organizations around the world are being challenged to reduce costs, provide more with less, while also improving the patient care system. The shortage of primary care physicians and nursing staff is forcing these already overworked professionals to work longer hours at the cost of their own health. The increasing longevity of human beings and increasing chronic illnesses and infectious diseases are changing the cost dynamics of healthcare. The complexity and competition in healthcare is further increasing with new approaches to healthcare and new businesses entering the market.
Analytics can help healthcare organizations harness big data and derive insights to help meet these demands. The information, insights, and predictions gained from analytics can be used by healthcare providers, hospitals, and the government to improve outcomes and deliver value for the people they serve.
Along with providing information to improve the patient care system, create better services, and streamline existing procedures, healthcare data analytics also provide financial and administrative data to healthcare organizations.
Healthcare business intelligence emphasizes data collection and analyses of costs and claims, research and development, and clinical data and patient behavior.
Data collection on costs and claims can assist managers and administrators in identifying the areas in need of smoother operations and smarter financial management. This data can also help understand the market and the demands of the consumers, which an organization can use to gain market share.
Likewise, data collection for research and development can help in better tracking, measuring, and analyzing diseases and illnesses, which can lead to innovative treatments and solutions. Analyses of clinical data helps healthcare providers determine the areas where service needs additional assistance and offer clarity regarding the effectiveness and success rates of treatments. Analytics also assists with the healthcare service providers in understanding the patients’ needs and reactions to services and treatments which will further help to improve outcomes.
Additionally, analytics helps the hospital administrators gain access to physician records and patient history, thus ensuring that the right doctor or professional is available at the right time for the best outcome.
Healthcare analytics is also helpful for insurance companies as they help track existing claims, clients, and premiums. This can help them monitor open claims, adjust policies, detect fraud, and provide more affecting pricing models for services.
Types of healthcare analytics
Any data relating to the health of an individual or a collective population is classified as healthcare data. Four major types of data analytics methods help in determining different aspects of healthcare:
- Descriptive analytics can be used for things like examining the positivity rate of a virus in a specific group of people over a certain time which can help in determining how contagious a virus is (the R0).
- Diagnostic analytics can be used in scenarios such as diagnosing an injury or illness of a patient based on their symptoms.
- Predictive analytics can be used to perform calculations that can predict the spread of a seasonal disease such as whooping cough by examining data from the past.
- Prescriptive analytics can be used to predict risk for developing future conditions based on a patient’s pre-existing conditions, and their compliance with drug-taking protocols. This means providers can implement specific, individualized preventative treatment plans with a greater understanding of the risks.
The data is gathered from a variety of sources, some of which include:
- Electronic health records (EHRs)
- Personal health records (PHRs)
- Electronic prescription services (E-prescribing)
- Patient portals
- Master patient indexes (MPI)
- Health-related smart phone apps
Uses of healthcare data analytics
Detecting fraud risk
Organized illegal schemes, deceitful practitioners, or even honest providers who make unintentional mistakes can cause healthcare fraud. This can be predicted through analytics by analyzing claim patterns across various insurance policy providers and by detecting duplicate and phantom billings.
Patient predictions
Analytics can provide information about the numbers of patients coming into hospitals daily and hourly, which can help predict the peak hours of patients' visits to hospitals. This can inform staffing requirements for healthcare providers so they can provide the best service while maintaining wage budgets and abide by proper nurse to patient ratio guidelines.
Evaluating and improving providers
Collecting patients' reviews on their experiences with their medical providers can help understand where practitioners can improve their behavior and attitude towards patients. Once the analytics identify the area where the practitioner may be struggling, these practitioners can be trained in alternative ways to interact with patients, creating a friendly, non-judgemental environment for the patients.
Analysis of electronic health records (EHRs)
Regular analysis of patients' digital health records, which includes medical history, allergies, tests results, and prescriptions, can help track a patients' wellbeing, alert practitioners, and send reminders if new tests are required. It can also track prescriptions to see if the patient is following the doctor's orders.
Predicting outbreaks
Healthcare analytics can help predict trends in the spread of viruses and illnesses, thus giving hospitals and healthcare providers adequate time to prepare. This can be done by collecting the data of positive cases in a certain area over a certain period, predicting the average time of the spread of the virus. This modeling has been used extensively throughout COVID-19, helping providers predict when there will be a surge in cases.
Developing new medications and therapies
Data analysis plays a vital role in the development of new and innovative treatments by using historical, real-time, and predictive metrics. This can help the experts identify the potential strengths and weaknesses of any trial, process, or medication.
Challenges and solutions with healthcare analytics
Healthcare data analytics can bring ground-breaking changes to the healthcare industry like reducing the cost of treatments, predicting outbreaks, and treating illnesses at an initial stage. The data is collected using various tools including electronic health records (EHRs), personal health records (PHRs), patient portals, medical insurance claims, health information exchange and more. When all the data is put together it can help make better predictions and design targeted interventions. However, with the data flowing in from a variety of sources, its proper implementation comes with a few challenges for healthcare organizations. Some of the major challenges and their solutions include:
Capturing and storing accurate data
Healthcare data is collected from different sources and in different formats. These formats can include structured data, papers, photographs, multimedia and personal health devices on the Internet of Things (IoT). In such a situation, capturing accurate, clean data and formatting it to use in a variety of frameworks becomes a challenge for healthcare organizations. While the data is collected by providers, public health experts, employers, and directly by patients, the variety of quantitative and qualitative data is recorded in a variety of formats. As a result, it becomes difficult to get an understanding of a patient’s well-being journey and see trends or patterns in their care.
Bifurcating and filing the data type from both internal and external sources in different formats can help create a clean, accurate and effective dataset. Better data capturing techniques can be created by data providers by organizing important data types on a project basis which will help in meaningful downstream analytics.
Keeping datasets up to date
It is likely that a patient’s health statistics will fluctuate over their lifetime. Also, there is always a chance that a patient changes their address, profession, or even their name a few times in their lifetime. This may lead to duplication and miscalculations. In such situations, the data providers need to ensure that the data is updated both automatically and manually so that the best quality data is available to help providers diagnose and treat the patient. This can be done by developing multidisciplinary groups that can separate the data, run duplicity tests, and update the files with the latest information.
Maintaining privacy and security
It is the responsibility of the medical administrators and professionals to keep the patient's personal details secure while improving their well-being and health. The personally identifiable information (PII) needs to be protected at all costs. Data security is of utmost importance in the healthcare industry, and the Health Insurance Portability and Accountability Act (HIPAA) prevents sensitive information about a patient from being shared without the patient's knowledge and consent. The HIPAA security rules have a series of security protocols for organizations that store protected health information like authentication protocols, control over access, and auditing. A number of malware, phishing assaults, and other cyber-attacks are always threatening digital data.
The digital data can be protected by installing up-to-date antivirus software, encrypting sensitive data, multi-level clearances, authentication, and more. At the same time, the staff who have access to sensitive data should be trained on the proper data security protocols and what to do if an issue occurs.
Data storage and documentation
While many organizations prefer in-house data storage for better control over security and access, the massive amount and the variety of healthcare data makes on-site servers incredibly expensive.
This problem can be solved with the creation of a digital document management system that can help healthcare organizations save money and time while also providing document security, centralized storage, and access control.
Updating staff on new technology
When a data analytics system is put in place, it becomes mandatory for staff of the healthcare organization to be trained on correct usage. This can be done by providing regular training and refresher courses to the staff. At the same time, a specialized technical team including data scientists and analysts should be established to maintain the system.
How to build an effective healthcare analytics strategy
There are four major points that should be considered while preparing an effective healthcare analytics strategy.
1. Collecting the right data
Healthcare data is generally unstructured and available in different forms. To start with, the data being used must be complete, accurate, and formatted correctly. This can be done using proper data management technology. This will allow the organization to use the data across different platforms and gain better and more accurate insights.
2. Eliminate bias
Real world biases can affect how data is recorded, especially qualitative data. It is critical that the data analytics strategy contains a method to identify and eliminate potential biases. This can be done by working closely with care equity experts and patients.
3. Ensure patient privacy
Data sharing among different organizations, healthcare providers, research centers, and others is a must to get the most out of the analytics. However, this needs to be done in accordance with data sharing regulations, keeping in mind the privacy of the patients whose data are being shared. This data should be anonymized when possible to protect patient confidentiality and reduce the risk of the data being misused.
4. Bringing in external expertise
While medical organizations collect huge amounts of data on a daily basis, making use of it in the best way may not be standard practice in hospitals and doctors offices yet. It has been suggested that organizations would benefit from the integration of data scientists and the different data management and analysis tools available.
While the healthcare industry does currently use healthcare analytics to some degree, in the coming days its application will become more common. Healthcare organizations across the globe have already started employing artificial intelligence, machine learning, and natural language processing to translate the available data and find new methods of data collection and processing. With the globalization of healthcare analytics, now is the right time for healthcare organizations to start implementing analytics for better results, interventions, and healthcare facilities.
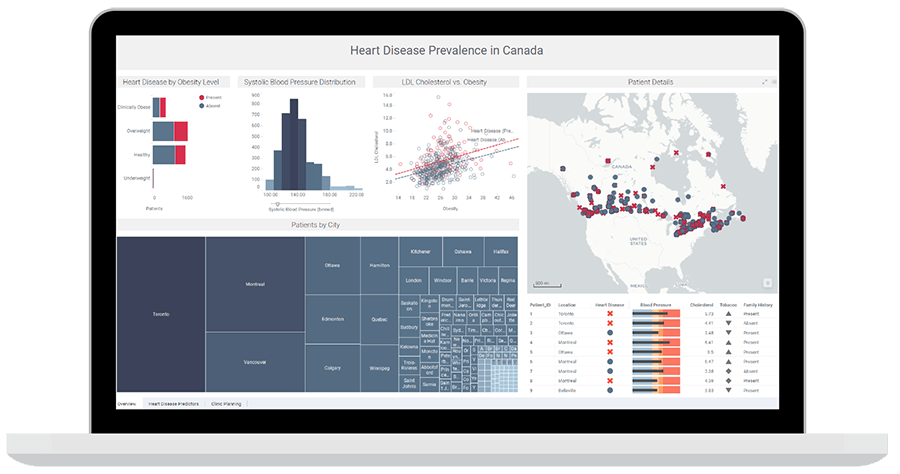