What is banking analytics?
Banking analytics refers to the use of artificial intelligence and machine learning being applied to customer data to make decisions in the banking sector. Data is analyzed, trends identified, and predictions made. This helps managers to make better business decisions.
How are banking analytics used by organizations?
Banking analytics is used to identify trends that help management in decision making. Assessing and measuring historical data makes it possible for behaviors to be identified and future trends predicted and used as the basis of future decision making. By utilizing data analytics tools and processes, the bank is able to make informed decisions that can prevent errors and improve efficiency.
Banking analytics is an important management tool that gives insight on current performance and highlights areas where improvement is needed. It can be difficult for banks to tell which areas need to be reviewed or improved without access to data backed metrics and predictive future outcomes. Banking analytics makes it possible for hidden performance issues to be identified and addressed.
While individual organizations will interpret and act on data outcomes differently, there are a number of standard ways that banking analytics is used.
Risk management
The major revenue stream for banks is selling loans, and this makes up the highest percentage of a bank’s income. However, despite the potential returns associated with these loans, it can also be an extremely risky venture for banks. There is the possibility of people failing to service their loans, resulting in losses for the financial institution. It is important for banks to have a way of vetting potential customers to determine their risk levels and decide whether to approve them or not.
Banking analytics tools are used by financial institutions to categorize their customers in accordance with their risk levels. Customers who have a lower risk level benefit from reduced interest rates as they pose little threat to these institutions. Those customers with lower credit ratings will be charged higher interest rates as they are deemed as risky borrowers. Potential borrowers deemed high risk may be denied loans altogether.
Measuring productivity
Another example of how banking analytics is used in the banking sector is measuring the productivity of the organization’s employees. Such measurements enable the banks to understand whether or not they have adequate human resources. Results from such measurements can lead to recruiting more employees if the current team is overworked. The analysis can also be used as the basis of reducing the workforce, especially where it has been identified that there are areas of over staffing.
Reducing wastage
When a bank is in the process of determining the level of wastage in its human resource, it adopts the use of analytics to enable them to identify areas needing improvement. One such analytics tool is the cost per teller transaction. The tool analyzes the amount of money that it costs the bank for each transaction that is executed by the tellers. The analysis is carried out across the entire branches over a given period of time. High values indicate that the bank is losing a lot of money either as a result of inefficiencies or human error.
The findings of this analytical tool are used to determine the most appropriate course of action that can be adopted in order to reverse the trend. In case the losses are a result of human error, training and development programs can be instituted in order to sensitize the employees about the identified errors and how to avoid them. In case the high cost of transactions is a result of system inefficiencies, a way of improving it should be identified.
Time management
Another example of how banking analytics can be used in real banking settings is by analyzing the amount of time it takes to serve a single customer from the initiation of a transaction to its conclusion. Teller transaction cycle time is a banking analytics tool that measures the amount of time a teller takes to complete a transaction. Customers generally prefer to be served within the shortest time possible and any delays in service delivery can result in complaints, dissatisfaction, and ultimately churn.
Promoting consistency
Enhancing consistency in the time taken to complete a transaction is also a way of promoting customer satisfaction. Inconsistencies in the time taken to complete a transaction means that there is an issue that needs to be addressed, such as ineffective technology, lack of on-site aids, and inadequate training programs for employees.
When the tool identifies that excessive time has been used to complete a transaction, there is a need for corrective measures to be taken to rectify the situation. In this way, the bank will be able to meet the expectations of their customers, leading to higher satisfaction ratings.
Measuring total deposits
Banks can also use analytics tools to measure the total number of deposits that have been received from their customers at any one given time. The data collected helps in determining the profitability of the different branches. Any branch that has a comparatively low number of deposits is an indication that something is amiss and that there may be a need for measures to be taken to reverse the trend.
Marketing targeting
One of the issues that can make a branch comparatively less profitable is the accuracy of targeting for marketing campaigns. If the branch has not created aggressive or targeted marketing strategies for loans, it is possible that their sales will be low as compared to those that have well-structured marketing campaigns.
Measuring customer satisfaction
Poor customer satisfaction rating is an issue that can lead to one branch performing poorly compared to others. In such instances, management should measure customer satisfaction and drill down to find where the problems are arising and then address those issues.
Identifying fraud
Fraudulent activity costs the banking industry billions every year. As technology changes, criminals are evolving too, creating more complex situations for the industry and increasing the need for banking analytics tools. Using banking analytics tools identifies trends early on and informs the business which steps need to be taken to address the theft promptly.
Challenges facing banking analytics
Huge data volumes
The biggest challenge facing banking analytics today is the vast amount of data that is collected, cleansed, and processed. Banks collect huge volumes of data on a daily basis from sources such as automatic teller machines, credit card purchases, credit processing departments, and online banking data. Not all of it is useful or accurate enough for analysis.
Conducting data cleansing can be incredibly time and resource intensive. Data is collected in a range of formats that does not easily facilitate analysis. It is estimated that over 80% of analysts’ time is spent sorting out the data and formatting in order it to be made compatible with the requirements of analysis tools.
This can be addressed by adopting a system that is able to adequately and quickly sort the data to determine what is useful for analysis. The system should be designed in such a way that it can identify useful data, and perform basic data cleansing.
Data fragmentation and silos
Data silos and fragmentation present serious challenges to the banking industry and pose a threat to banking analytics’ accuracy and efficiency. Data relating to different transactions is usually stored in different departments, which makes consolidating it a cumbersome undertaking. This means that either banks will not be able to use all relevant information, or a lot of time and effort will be taken trying to consolidate data. Whichever the case, the quality of the analysis will be affected, and this can lead to poor decisions being made for failing to factor in important data.
The problem of data fragmentation can be solved by using a system that is able to incorporate all the departments into one system. Most of the traditional enterprise resource planning systems used in the banking industry utilize different software for different departments. As such, customer data is fragmented across different datasets making it difficult to retrieve and make a comparison.
Use of advanced systems such as cloud enterprise resource planning can store information from different departments into one dataset. In this way, it is easier for the system to extract and compare and process data as needed.
Use of outdated systems
The use of outdated systems in the banking sector is another challenge facing data analytics in the sector. These legacy systems are not designed to meet the emerging need for data analysis. As such, their functionality is limited to what they were designed to analyze when they were developed. In addition to this, these systems are not designed to deal with massive data that comes from many different sources.
For example, legacy systems that most banks use were developed when internet and mobile banking were in their infancy. They are simply not designed to accommodate the volume and type of data from banking apps, online banking, and apps such as GPay and Apple Pay. Mobile and internet banking generate huge amounts of data that may not be captured by the traditional systems during the analytics process.
The problem of using out-dated systems can be addressed by adopting the use of modern cloud-based systems. There are a range benefits of cloud-based systems–upgrades are completed automatically, data is accessible from all geographic locations, and they assist in avoiding data fragmentation and silos. The use of a cloud-based system in banking analytics contributes to a more seamless working environment that ensures better data processing.
Data privacy and security
With great data comes great responsibility. Particularly in the banking sector, privacy and security are vital; money is important. There are many facets to this problem.
Data security from outside threats
While putting data in the cloud is an attractive, scalable, and easy option rather than storing information physically on site, it means there are more opportunities for hacking or interception of data. Banks must have stringent security controls to protect against cyber attack.
Data security from inside
There need to be stringent protocols in place so employees cannot access data they do not need. Access of banking records should be strictly controlled.
GDPR breaches
For both internal and external threats, non-compliance with data security means that organizations are liable for large fines, penalties, and loss of trust from their customer base. Additionally, before using client data, the customer must be asked for permission.
Data and banking analytics in the future
With the expansion of the Fintech industry, the traditional banking sector has been forced to evolve. The ease of use of data and information technology is what consumers demand, and the older methods of banking are falling out of use.
Modern banks must have an analytics department. Hiring a range of specialists such as data scientists, statisticians, and those experienced with analytical tools and software is essential to ensure best data and analytics practices are followed. These people must be supported and assisted by banking experts and leaders in their departments.
In order to remain relevant and retain market share, banks must adapt their practices. Part of this is using banking analytics to provide better service, increase market share with more effective advertising, and differentiate themselves from their competition.
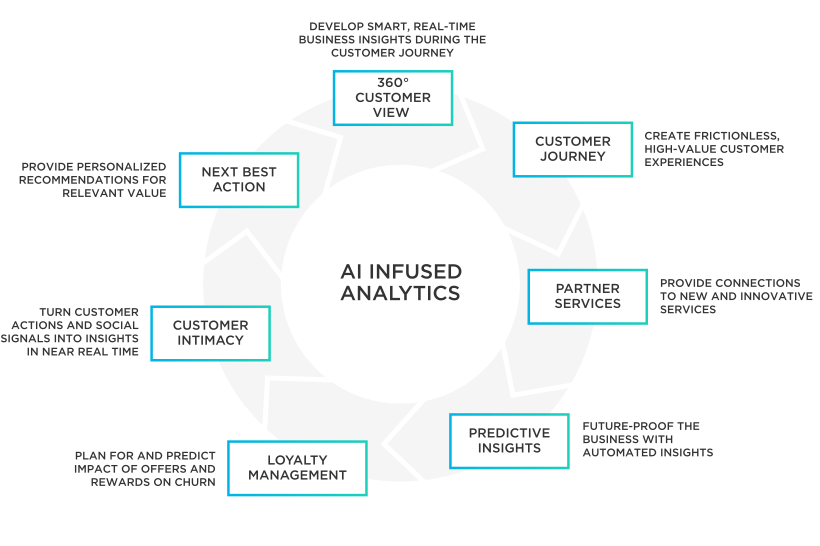