What is factor analysis?
Factor analysis is a statistical technique that reduces a set of variables by extracting all their commonalities into a smaller number of factors. It can also be called data reduction.
When observing vast numbers of variables, some common patterns emerge, which are known as factors. These serve as an index of all the variables involved and can be utilized for later analysis.
Factor analysis uses several assumptions:
- The variables’ linear relationships
- Absence of multicollinearity
- Relevance of the variables
- The existence of a true correlation between factors and variables
Therefore, it becomes a statistical technique used to see how a group shares a common variance. While it is mostly used in psychological research, it can also be applied in areas like business and market study to understand customer satisfaction or employee job satisfaction and in finance, to study the fluctuation of stock prices.
Features of factor analysis
While studying customer satisfaction related to a product, a researcher will usually pose several questions about the product through a survey. These questions will consist of variables regarding the product’s features, ease of purchase, usability, pricing, visual appeal, and so forth. These are typically quantified on a numeric scale. But, what a researcher looks for is the underlying dimensions or “factors” regarding customer satisfaction. These are mostly psychological or emotional factors toward the product that cannot be directly measured. Factor analysis uses the variables from the survey to determine them indirectly.
When a researcher assumes these variables from the survey, they are condensed into one or more factors. Some of the methods used to extract these factors could include:
Principal component analysis
This is the most commonly used method. The first factor is extracted by determining the maximum variance. This variance is then removed and is replaced by the factor. The second factor is then determined by the next highest variance, and the process continues until there are no more variances.
Common factor analysis
In this method, the factors are extracted from commonly-occurring variances and do not include the unique variances of all the variables.
Image factoring
Based on the correlation matrix, this process uses predicted variables using the OLS regression method.
Once the factors are extracted, the questionnaire’s score is assumed to be related to the factors in a linear manner. The margin of error is also taken into consideration, along with all the factors to the equation.
Types of factor analysis
There are essentially two types of factor analysis:
- Exploratory Factor Analysis: In exploratory factor analysis, the researcher does not make any assumptions about prior relationships between factors. In this method, any variable can be related to any factor. This helps identify complex relationships among variables and group them based on common factors.
- Confirmatory Factor Analysis: The confirmatory factor analysis, on the other hand, assumes that variables are related to specific factors and uses pre-established theory to confirm its expectations of the model.
Assumptions of factor analysis
Factor analysis makes use of several assumptions in order to produce the outcomes:
- There will not be any outliers in the data.
- The sample size will be greater than the size of the factor.
- Since the method is interdependent, there will be no perfect multicollinearity between any of the variables.
- When in a sequence of random variables, all the variables have the same finite variance, known as being homoscedastic. Since factor analysis works as a linear function, it will not need homoscedasticity between variables.
- There is the assumption of linearity. This means that even non-linear variables can be used, but once transferred, they become linear variables.
- There is also the assumption of interval data.
How factor analysis is used
Business marketing
In a business model, factor analysis is used to explain complex variables or data using the matrix of association. It studies the interdependencies of data and assumes that complex variables can be reduced to a few important dimensions. This is possible because of some of the relationships between variables and their dimensions. The attribute of one variable might sometimes be the result of the dimension of another. It breaks down the initial rating, using statistical algorithms on various components and uses these partial scores to extract various factors.
Automotive industry
The use of factor analysis in the automotive industry was mentioned as far back as 1997 in an article by Professor Emeritus Richard B. Darlington of Cornell University. He explained how a study could be used to identify all the variables that apply to the decision-making of purchasing a car—size, pricing, options, accessories, and more. The study could then be used to arrive at a few key variables that actually close a purchase decision. Automotive dealers can then tailor their offerings to cater to the market.
Investing
The key to a productive investment portfolio is diversification. To ensure a diverse portfolio, investment professionals use factor analysis to predict movement across a wide sector of industries and provide insights on factors that may be under the radar. For example, the average portfolio contains stocks of industries like technology and commodities. A look at the rise in stock prices of a related industry, like oil, will give investment professionals a good idea on what to sell and retain.
Human resources
There are many factors that go into a company’s hiring process. With statistics, human resource professionals will be able to create a comfortable and productive working environment. Several variables can be compared and analyzed to see which combination in terms of the number of team members, varied skill sets, and contractual or in-house talent works, improving the overall functioning of the organization.
Restaurants
For restaurants, factor analysis can be used to understand demographics and target diners in the creation of menus. A fast-food restaurant opening next to a university campus will have to plan its menu differently than if it was placed in a high-end shopping location. Factors such as surrounding competition, foot-traffic, age-groups, and location all determine success.
Education
When hiring teachers and deciding on a curriculum for the school year, factor analysis plays a huge role. It is used to determine classroom sizes, staffing limits, salary distribution, and a wide range of other requirements necessary for the school year to run smoothly.
Challenges and solutions of factor analysis
While factor analysis is a useful tool for business research and analysis, there are a few challenges to keep in mind in order to ensure the right results. The result entirely depends on the ability of the researcher to gather the right set of variables associated with the business and the product. Neglecting even a small detail might result in the wrong value of the procedure.
If the observed variables for a particular item are similar to each other but distinct from other items, the algorithm might consider this as a single factor to those items, which could result in inaccurate analysis results. Knowledge of the item and its theory is important in naming factors accurately. Even dissimilar variables might have dependencies for no reason.
Gathering information using an accurate survey is the key. Besides the knowledge of the product and its theory, it is also important to know its market.
Research and developments in the field of factor analysis continue to contribute to making more informed decisions in various sectors. Continuous refinement in confirmatory factor analysis techniques makes this one of the most important decision-making tools for every industry in the future.
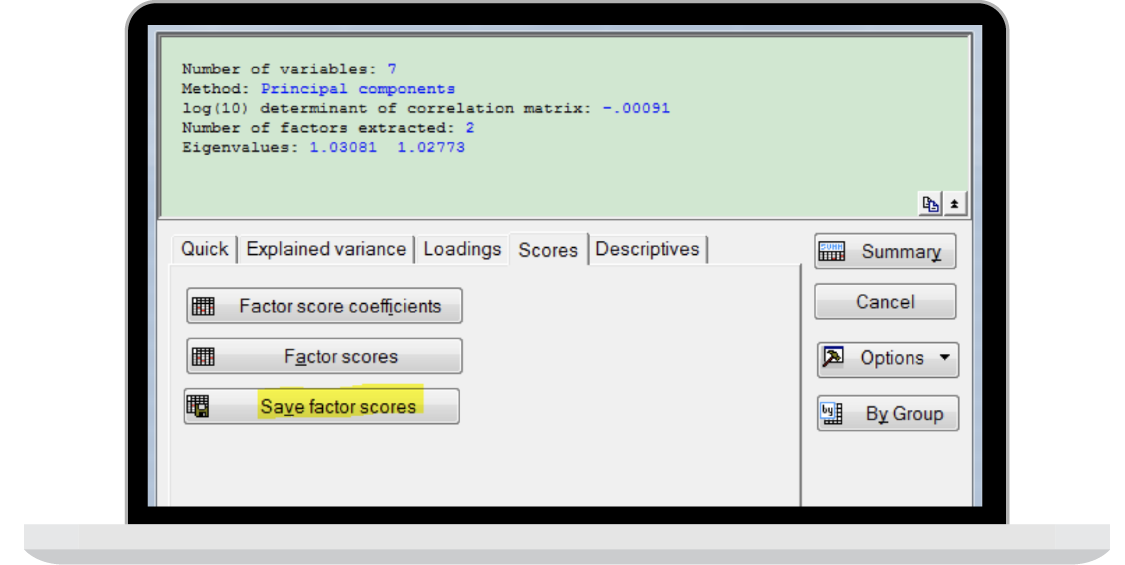