What is statistical process control?
Statistical process control (SPC) monitors manufacturing processes with technology that measures and controls quality. SPC triggers various machines and instruments to provide quality data from product measurements and process readings. Once collected, the data is evaluated and monitored to control that process.
Statistical process control is a simple way to encourage continuous improvement. When a process is continuously monitored and controlled, managers can ensure that it works at its full potential, resulting in consistent, quality manufacturing.
The concept of statistical process control has been around for a while. In 1924, Bell Laboratories employee, William A Shewart, designed the first control chart and pioneered the idea of statistical control. This quality control process was extensively used during World War II in facilities for ammunition and weapons. Statistical process control monitored the quality of products without compromising on safety.
Following the war, the use of statistical process controls slowed down in America but was picked up by the Japanese--who applied it to their manufacturing sector and continue to use it today. In the 1970s, statistical process control came back into use in America to rival products from Japan.
Today, the worldwide manufacturing sector uses statistical process controls extensively.
Modern manufacturing companies have to deal with constantly fluctuating prices of raw materials and a great deal of competition. Companies cannot control these factors, but they can control the quality of their products and processes. They need to constantly work on improving the quality, efficiency, and cost margins to be a market leader.
Inspection continues to be the key form of detecting quality issues for most companies, but its efficacy is debatable. With statistical process control, an organization can shift from being detection-based to prevention-based. With the constant monitoring of process performance, operators can detect changing trends or processes before performance is affected.
An overview of statistical process control tools
In total, 14 quality control tools are used in statistical process control, which are divided into seven quality control tools and seven supplemental tools:
Quality control tools
Cause-and-effect diagrams
Also known as the Ishikawa diagram or the fishbone diagram. Cause-and-effect diagrams are used to identify several causes of any problem. When created, the diagrams look like a fishbone, with each main bone stretching out into smaller branches that go deeper into each cause.
Check sheets
These are simple, ready-to-use forms that can be collected and then analyzed. Check sheets are especially good for data that is repeatedly under observation and collected by the same person or in the same location.
Histograms
Histograms look like bar charts and are graphs that represent frequency distributions. They are ideal for numbered data.
Pareto charts
These are bar graphs that represent time and money or frequency and cost. Pareto charts are particularly useful to measure problem frequency. They show the 80/20 Pareto principle: addressing 20 percent of the processes will resolve 80 percent of the problems.
Scatter diagrams
Also known as an X-Y graph. Scatter diagrams work best when paired with numerical data.
Stratification
This is a tool to separate data that simplifies pattern identification. Stratification is a process that sorts objects, people, and related data into layers or specific groups. It is perfect for data from different sources.
Control charts
These are the oldest, most popular statistical process tools.
Supplemental tools
Data stratification
A slight difference from the stratification tool in the quality control tools.
Defect maps
These are maps that visualize and track a product’s defects, focusing on physical locations and flaws. Every defect is identified on the map.
Events logs
These are standardized records that record key software and hardware events.
Process flowcharts
Process flowcharts are a snapshot of steps in a process, displayed in the order they occur.
Progress centers
When decisions need to be made, progress centers are centralized locations that allow businesses to monitor progress and collect data.
Randomization
Randomization is the use of chance to assign manufacturing units to a treatment group.
Sample size determination
This tool determines the numbers of individuals or events needed to be included in the statistical analysis.
Benefits of statistical process control
Statistical process controls have a range of benefits:
- Reduced wastage and warranty claims
- Maximized productivity in a manufacturing unit
- Increased operational efficiency
- Reduced need for manual inspections
- Enhanced customer satisfaction
- Controlled costs
- Improved analytics and reporting
How to use statistical process control
As with any new process, the first step to using statistical process control is evaluating where the manufacturing business is facing waste or performance issues. These could be related to reworking products, wasting entire ranges of products, or long inspection times. A company benefits by applying statistical process control systems to these trouble areas first.
Statistical process control doesn’t always relate to factors like expense, time, or production delays, depending on the need. When implementing new processes, a cross-functional team will identify the critical aspects of either the design or the process that needs addressing.
This is done in the course of a print review or an exercise known as design failure mode and effects analysis (DFMEA) exercise. This exercise’s data is collected and monitored for critical characteristics in the following process:
Collection and recording of data
Statistical process control data is collected in two ways: first as measurements of a particular product and second as process instrumentation readings.
Once recorded, this data is tracked with several kinds of control charts, specific to the type of data collected. Using the appropriate chart is imperative to gaining useful information. Data is either the continuous variable or is attribute data. Collecting and recording data can be individual values or can be the average of a set of readings, depending on what the organization needs.
With variable data for individual values, a moving range chart is used. If data is recorded in sub-groups of eight or less, then an X-bar R Chart is used. If the subgroup value is higher than eight, then the X-bar S chart is used.
With attribute data, an A P chart is used to record how many defective parts are within a set of parts. An A U chart helps record how many defects are in each specific part.
Control charts: Referred to as X-bars and R Charts. The X-bar refers to the “mean” of variable x. Range charts are representative of the variations within subgroups. Range here refers to the difference between the highest value and lowest.
Here are the steps to build an X-bar and R chart:
- Begin by designating a sample size as “a.” A common number is four or five, but the sample has to be below eight. Settle on the frequency of sample measurements in this step.
- Begin gathering an initial sample set. A rule of thumb is 100 measurements in a set of four, resulting in 25 data points.
- Start by calculating the average value for every one of the 25 groups of each of the four samples.
- Next, compute the range in the same way for each of the four measurement samples. The difference between the highest value and lowest in each set is the range.
- The next step is to calculate the average of the averages. This is for the X-bar and is represented by a solid line.
- Next, calculate the “R” value averages, represented by a center-line.
- The next step is to work out the upper and lower control limits for both the X-bar and the R charts.
- With the chart in hand, the technician will continue to measure several samples, adding the values, and then calculating the average. These values are recorded in the X-bar chart. Sample measurements must be recorded at fixed intervals, along with the date and time, to track process stability. If any unique issues arise, they have to be adjusted into the process to maintain stability.
X-bars and R-charts are some examples of the kinds of control charts used to monitor and improve processes.
Analyzing the data
During analysis, all data points recorded on the control chart have to be within control limits, as long as there are no special causes. Common causes result in data points falling within control limits, but special causes tend to be outliers. To classify a process as being in statistical control, there should be no outliers on any chart. When a process is in control, it will not have any identified special causes and all the data will fall between control limits.
However, there are examples of common cause variation:
- A change in material properties
- Seasonal related changes to temperature or a change in humidity levels
- Regular wear and tear of machines or tools
- Variations in operator related settings
- Regular measurement variations
Special causes fall outside the purview control limits and can indicate a massive change in the process. These variations can include things like:
- Failed controllers
- Incorrect equipment adjustment
- Changes in measurement systems
- Shifts in process
- Malfunctioning machines
- Resource material properties that fall out of the purview of a design specification
- Broken tools
- Operator inexperience
While monitoring processes with statistical process control charts, all data points are constantly verified to check if they are remaining within control limits. They will watch out for any changes in trends or abrupt changes in processes. If a special cause is identified, necessary action is taken to determine the cause. Next is to remedy it and allow the process to come back to statistical control.
Besides these variations, there are other data point variations that fall within control limits and should be investigated:
- Cycles where seven data points or more end up toward one side of the process center line
- A change in the usual expanse of data. This could be where several data points end up close to each other or far apart
- When a new trend arises, with seven data points or more, constantly going up or down
- Changes in the spread of data that are clearly either above the normal mean or below it
Disadvantages with statistical process control
As with any process, there are some disadvantages to statistical process control:
Time requirements
While the emphasis of statistical process control is on early detection, implementing the system in a manufacturing set up can take a long time. Additionally, the process of monitoring and filling out charts is time consuming. Since the system has to be integrated into an existing framework, training of personnel is required which takes time.
Cost considerations
Statistical process control is also an expensive affair and requires that a company signs a contract with a service provider and invests in training resources and materials.
Quality measurements
A problem with statistical process control is that it detects when there is non-conformance in the process protocol, but it does not say how many products may be defective up until that point.
The future of statistical process control
By stabilizing its production process, an organization can reduce the number of variations in productivity, benefitting both the consumer and the company. While the consumer gets a safe and well-tested product, and the company benefits by reducing the costs of production and any embarrassment that arises from a defective product.
As machine learning and artificial intelligence advance, the abilities of statistical process control will increase. This will lead to bigger gains for the manufacturer, increased competitive advantage, and satisfied customers.
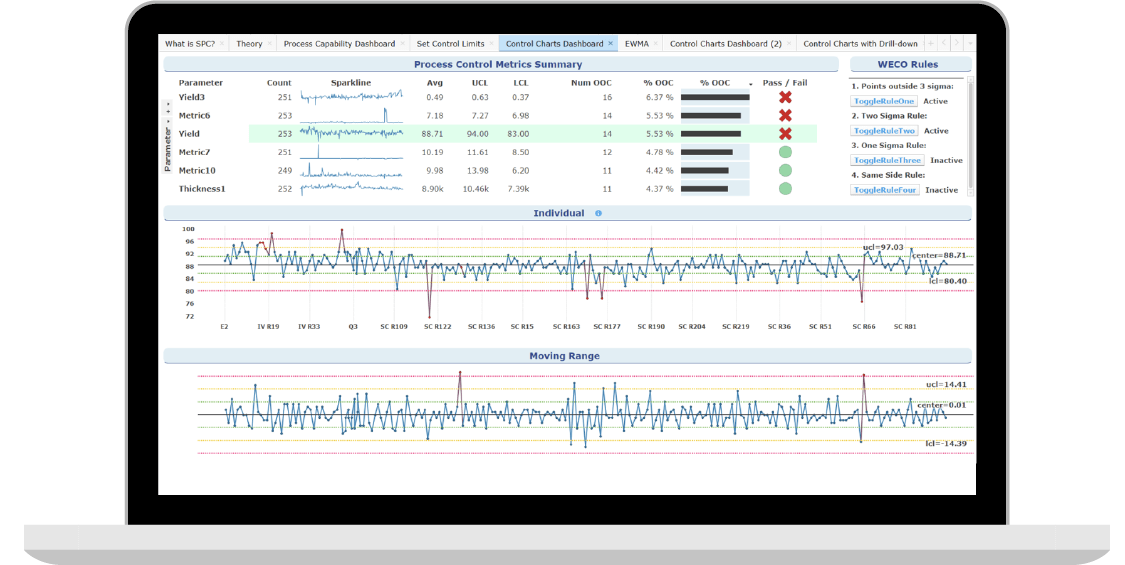